A best management practice results in maximum yield balanced against minimum inputs, a small environmental footprint and overall production system sustainability. But knowing which management decisions are best is complicated.
Recent technological advances, however, are making it easier as efficient and inexpensive on-farm research and data collection are helping farmers make the most of superior data-driven, decision-making tools and software. These new tools should help farmers to easily optimize precision management decisions in real-time.
Most farmers are familiar with combine-mounted GPS and yield and protein monitors that allow them to collect location-specific data on yield and grain protein concentration on-the-go. Other technologies are also readily available. Those include remote sensing imagery, such as low-cost, high-resolution satellite imagery [e.g., RapidEye, PlanetScope, SkySat, and free Google Earth Engine (https://earthengine.google.com/)], and aerial imagery (multispectral cameras mounted on airplanes or drones) provided by local companies.
Soil properties, including electrical conductivity, pH, and soil organic matter (SOM), can be mapped at high-resolution by several types of on-the-go soil sensors (e.g., Veris machine). These indices can provide insight into the spatial and temporal variation of crop properties.
Some farmers have installed weather stations in their own fields and WSU AgWeatherNet provides statewide weather data and weather-related decision-support tools (https://weather.wsu.edu/). Free weather station data or 4-km-grid resolution and radar maps of daily weather conditions are also available from the National Climate Data Center (http://www.ncdc.noaa.gov/cdo-web/). Some business entrepreneurs are marketing weather systems, such as the aWhere Platform and WeatherHawk. They claim their data can help farmers react more quickly and more profitably to changing weather conditions.
In hilly fields, the 3D Elevation Program (3DEP) (https://viewer.nationalmap.gov/basic/) can be an extremely useful data layer for calculating indices such as slope, aspect, topographic wetness index, total solar radiation, and total terrain surface curvature. Entrepreneurs are also taking advantage of these new technological resources to provide tools capable of generating zone maps and variable-rate fertilizer prescriptions.
For farmers wondering how to use this vast amount of data (Big Data), agribusinesses worldwide are also selling “data-driven” management advice software for “smart farming”, including Ag Leader’s® SMSTM, SMART! Fertilizer Management, Farmer’s Edge’sTM Smart Solutions, DuPont Pioneer’s EncircaSM, and Yara International’s Adapt-N. The maps and prescriptions from these programs are often created based on historical remote sensing imagery, yield maps, and other site-specific information.
But the technologies do not come without drawbacks. Here are three examples of problems associated with these methods:
(1) Numerous studies have provided empirical evidence rejecting the yield-goal-based approach (used in many of the above scenarios) due to its over-simplification of agricultural production complexities within the larger contexts of agroecological and socioeconomic systems. Nitrogen (N) management is an example. The yield-goal method estimates N fertilizer rate using a mass balance approach that adjusts unit N requirement (UNR) (e.g., 2.7lbs N/acre/bushel soft white winter wheat or 5.7 lbs N/acre/100 lbs winter canola seeds) for yield based on soil N supply and N fertilizer use efficiency (NUE) under non-N limited conditions. UNR, however, can vary substantially within- and across-fields due to differences in annual rainfall, amount of rainfall per event, soil type, soil drainage class, or drain tile system. Crop rotation, tillage, and other management factors also influence UNR. In other words, many diverse factors lead to UNR variability in different locations within a single field and across fields. Thus, uniform UNR used in current methods neglects site-specific spatial variability of UNR.
(2) Soil N supply varies substantially in any location from year-to-year, depending on type and amount of plant residue, SOM content, proportion of different SOM pools, soil pH, soil management practices, and annual weather conditions.
(3) Weather contributes the most to yield and quality variability across years. Previous studies have found that the chance of the right rate being the same at a given field location across multiple years is very small. Current use of average yield or vegetation indices to determine N rate neglects this temporal variability. Dr. Bill Pan [Washington State University (WSU)] found that spring canola’s UNR varied from seven to 17 kg N/kg seeds across 12 site-years. Using the average of seven to 17 for all fields across Washington (WA) does not constitute “precision” or “site-specific” management.
Adapt-N is one of the best decision-making tools because it uses sophisticated analytical methods and modeling that consider all spatial and temporal variables that can significantly affect crop N response. However, Adapt-N was developed for corn. Adopting this system for Washington wheat farmers would require proper calibration using local data and world market prices for protein premiums.
Other commercial decision tools, including Bayer’s FieldView Pro and DuPont-Pioneer’s Encirca, rely on crop growth models to generate recommendations. Many researchers have concluded that current crop growth models “are in need of significant improvement before they are capable of being sufficiently reliable for use to predict management practices on a field” (Antle, 2019).
Capturing within- and across-field spatial and temporal variability to reduce uncertainties in current precision agriculture methods requires large amounts of on-farm, field-specific data collected across many years. With big data and advanced cyberinfrastructure and computational approaches, scientists from different disciplines can now collaboratively generate knowledge to deliver specific information from those fields about those fields.
This information can be used to better understand crop yield and quality responses to inputs under different field and weather conditions. For example, we can calculate how output (e.g., wheat yield, protein concentration, test weight) changes in response to variable nutrient application rates under various field management practices (e.g., tillage, crop rotation, residue management), field characteristics (e.g., topography; micro-climate; soil chemical, physical, biological properties), and weather (e.g., rainfall, extreme temperature fluctuations, growing degree days).
Consequently, knowledge derived from big data can be used to develop user-friendly software for farmers and consultants to create variable rate precision strategies with greater confidence. A transdisciplinary, multi-state team from WSU, the University of Illinois, Cornell University, Purdue University, Montana State University, the University of Nebraska, Louisiana State University, Iowa State University, and Ohio State University are currently leading efforts to make real smart farming come true. Which will ultimately help Washington wheat farmers to know exactly which Best Management Practices are really best.
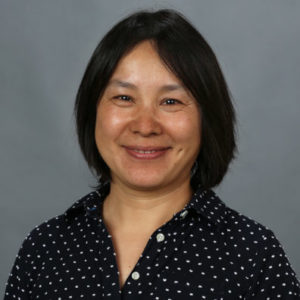