By Zhiwu Zhang
Artificial intelligence (AI) comes to every corner of our life. It is not only in the automatic driving cars on the roads, but also coming to our wheat fields and inspection stations to improve production.
Stripe rust, caused by Puccinia striiformis f. sp. Tritici, is one of the most devastating diseases of wheat and causes large-scale epidemics and severe yield loss. Applying fungicides during early epidemic development is crucial to controlling the disease, but is often challenged by resource-limited human visual scouting.
In the U.S. Pacific Northwest alone, growers spend over $10 million annually on fungicides for temporary solutions. Last year, I reported in Wheat Life Magazine how artificial intelligence was used to develop a computer vision system named RustNet (previously called Rooster) to help farmers use drone and phone images to detect wheat stripe rust at its early stage for timely fungicide applications.
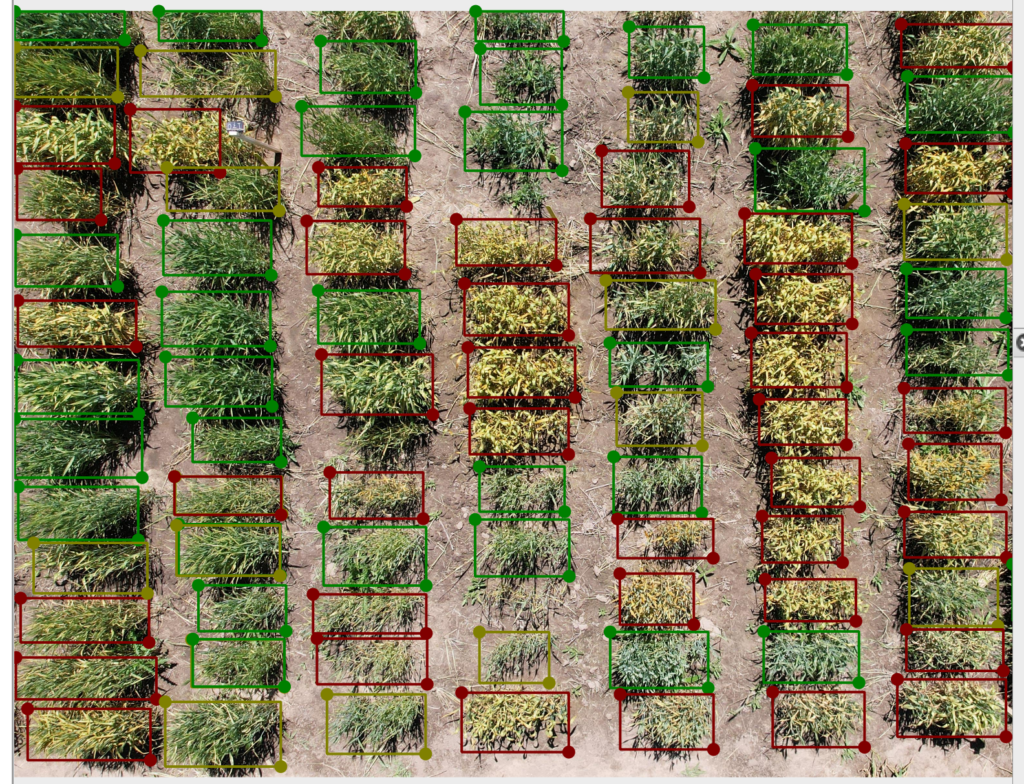
The technology was latter published by a peer reviewed journal, Computers and Electronics in Agriculture, in April 2023. An independent validation on field images from Germany achieved accuracies over 80%. RustNet is freely available at https://zzlab.net/RustNet.
Fungicide application implies a heavy financial toll and environmental footprint. Developing resistant cultivars is the most effective long-term solution.
Revolutionizing wheat cultivar development
Resistance evaluation of tens of thousands of breeding lines in experimental fields currently relies on visual observation of disease symptoms, which is labor-intensive, inefficient, subjective, and prone to error. The low efficiency limits the ability to develop resistant cultivars.
Drone images have the potential to enhance efficiency and accuracy. Our results from winter wheat stripe rust nursery fields suggested that breeding lines with high and low severity scores can be identified with accuracy above 90%. The breeding lines with high severity are framed with red and low severity with green in the picture below.
Although the system is currently still having difficulty differentiating the lines with moderate severity, the system can help breeders quickly find the targets and ignore the lines with problems.
Precision grain inspection
The wheat industry contributes over $1 billion to Washington state’s economy, with more than 80% of the wheat being exported overseas. Club wheat, a type of soft white wheat, commands a trade premium of up to $1.50 per bushel.
Club wheat is specialized for low gluten strength, clear separation between bran and endosperm for milling, and low water absorption. These properties place club wheat in high demand as an ingredient to mix with common wheat to produce special products such as sponge cakes.
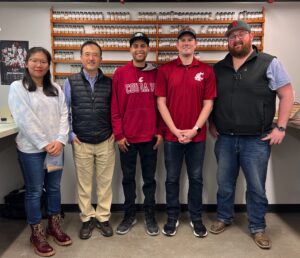
“Club wheat is used for blending with common soft white wheat to create special Western White products,” said Scott Steinbacher, program manager for the Warehouse Audit Program and the eastern regional manager for the Grain Inspection Program, both part of the Washington State Department of Agriculture (WSDA).
In 2022, Washington’s wheat production reached 144 million bushels, with club wheat accounting for 5% to 10% of the total. Due to grain inspection limitations, approximately 5% of club wheat is over-blended into common soft white wheat.
“The Washington grain industry potentially loses about a million dollars annually,” said Ben Barstow, Washington Grain Commission chairman.
“Currently, the identification of club wheat grains relies on trained inspectors based on visual characteristics, especially for certain club wheat varieties,” said Joshua Duncan, WSDA commodity inspection supervisor in Colfax, Wash. The human approach is subject to human errors and limited to the resolution of human eyes.
“The computer vision system we developed currently achieved 80% accuracy to differentiate club wheat from common wheat”, said Meijing Liang, a Washington State University Ph.D. graduate student in my lab.
Our team is working closely with the grain inspection station in Colfax to extend the potential applications, such as identifying hard white wheat as a contaminant in soft white or identifying mixtures of hard red spring in hard red winter.
This article originally appeared in the November 2023 issue of Wheat Life Magazine.
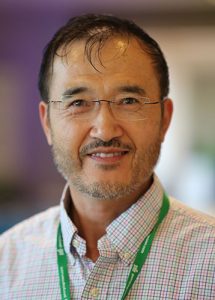
Zhiwu Zhang, Ph.D.
Zhiwu Zhang is an associate professor at Washington State University. His research interests include phenotyping by using hyperspectral images and remote sensing images from satellites and drones. He also works on innovative statistical methods and computing tools for gene mapping and genomic selection, along with integration of high throughput genotyping, statistics, and machine learning to solve problems in plant breeding. Read more about Dr. Zhang.