By Arron Carter
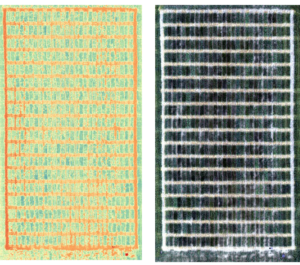
If you have recently been to a field day or heard me talking on the breeding program, you will have heard me talk about our work on high-throughput phenotyping. This is a term we use to generalize the work done on collecting images and other spectral data to inform us about the breeding lines we are evaluating and their overall plant health status. I like to say the images give us information about the plant that the eye can’t typically see. We can estimate water content, photosynthetic efficiency, transpiration rates, and canopy temperature, all traits which I can’t normally estimate with my eye when looking at a plant, but traits we think are important to developing new cultivars that will maintain high yield in the face of climate variability.
When I started at Washington State University (WSU) 13 years ago, genomic information was very hard to come by. Currently, technology has advanced sufficiently that genomic information can be quickly gathered on every line in the breeding program. This has resulted in utilizing statistical models, which were first developed for animal breeding, to predict future performance of current breeding lines. Using historical performance from thousands of lines within the breeding program, for which we have phenotypic and genotypic data, we can estimate future performance of new lines based on their genetic composition alone. What we now need is better phenotypic information to go into these prediction models.
While our past research has demonstrated that these prediction models can, with high accuracy, predict performance of lines regarding end-use quality and disease resistance, it has been harder to predict complex traits like grain yield. With so many traits like tiller number, spike size, number of kernels per spike, etc., making up final grain yield, it is hard to make predictions without collected data on all these correlated traits, something that would be very difficult to do on thousands of lines. Furthermore, yield has a complex interaction with the environment that it is being grown in, and variables such as rainfall, temperatures, soil conditions and many more also directly affect final grain yield. With all these complex interactions, more accurate estimations of parameters going into final grain yield are needed to maximize genomic prediction of this trait.
Our winter wheat breeding program has been using sensors to help understand grain yield since 2015. Over time, we have begun to understand what spectral traits are highly correlated with yield and when that data needs to be collected. These sensors have now been placed on drones, which allow us to image hundreds of breeding plots within a few hours. Data can then be extracted from these images to give us an understanding of how a plant is responding to different environments with regards to the previously mentioned correlated traits. We can now add spectral traits into our genomic prediction models to improve prediction accuracy with regards to grain yield and grain yield stability over time.
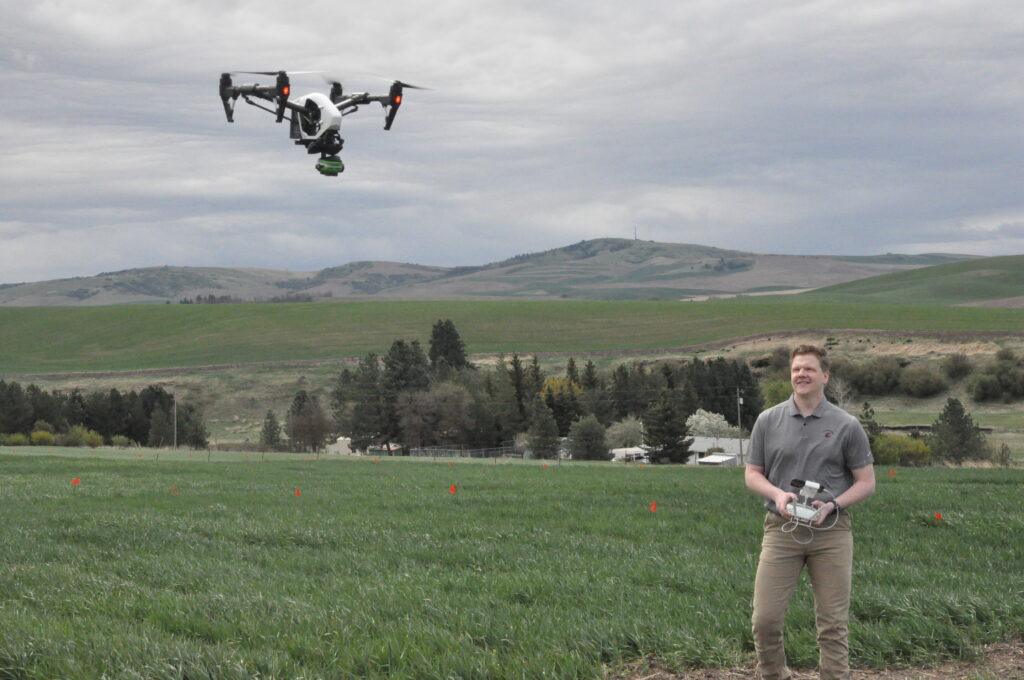
We know that we can improve genomic prediction of yield when we combine sensor data with other agronomic traits collected in the breeding program. Although an improvement, we still feel there is accuracy to gain. This comes in with weather information. We recently installed weather stations, in collaboration with METER Group Inc. (similar weather stations are part of the WSU AgWeatherNet system), to gain a better understanding of the environment the plants are growing in. Weather data will better help us understand temperature and rainfall patterns, as well as available soil moisture content, which will better help us understand grain yield at every location. As we better understand the environmental conditions that lead to final grain yield of each breeding line at each location, we hope to be able to incorporate this data into more complex genomic prediction models to better predict future performance of lines across environments. We currently do this by growing every line at as many locations as possible. So, if we can start predicting performance of lines in locations where we have not yet tested them, we should be able select more broadly adapted lines for trialing.
So where does the future of plant breeding go with this information? The Internet of Things (IoT) is a group of objects with sensors, software and processing ability, all connected to exchange data with other systems and devices over a communications network, like the internet. The best way to think about this is with the concept of a “smart home.” Today, you can have appliances, light fixtures, thermostats and security systems all connected and controlled through a communications device, like your cell phone. Transitioning this concept to plant breeding, soon we will have “smart fields.” Devices can be placed in fields with sensors that can monitor plant growth, disease, stress and other parameters, returning this data to the user in real time. As a plant breeder, I can have continuous data on plant growth, when diseases come into the field and which plants are affected, and at what time in the plant’s growth cycle is stress occurring. So instead of going out and flying drones at every field location, the IoT sensors will be placed in fields and provide continuous data for monitoring.
While the IoT concept is still in development, genomic prediction using high-throughput phenotyping and weather data is becoming routine selection within our breeding program. This prediction allows us to remove breeding lines that, based on genetics, will have a low potential of becoming a high yielding cultivar, allowing us to direct our resources to testing lines with higher genetic potential. This is all being done to release cultivars faster and with more confidence that they will be able to have high performance across multiple locations and in the face of annual climate variation.
This article originally appeared in the August/September 2022 issue of Wheat Life Magazine.
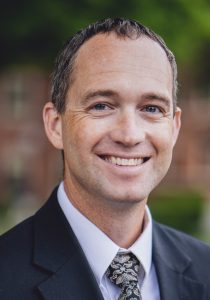
Arron Carter, Ph.D.
Arron Carter, Ph.D., holds the O.A. Vogel Endowed Chair of Wheat Breeding and Genetics at Washington State University. His research is directed toward breeding improved wheat varieties for cropping systems in Washington state that incorporate diverse rotations and environments. His research goal is to release high-yielding, disease resistant varieties with good end-use quality that will maintain profitability and reduce the risk to growers. New varieties are developed using a combination of traditional plant breeding methods, molecular marker technology, and biotechnology. Read more about Dr. Carter.